Contact us and see what NetOwl can do for you!
Name Matching Helps Protect Insurance Companies from Money Laundering
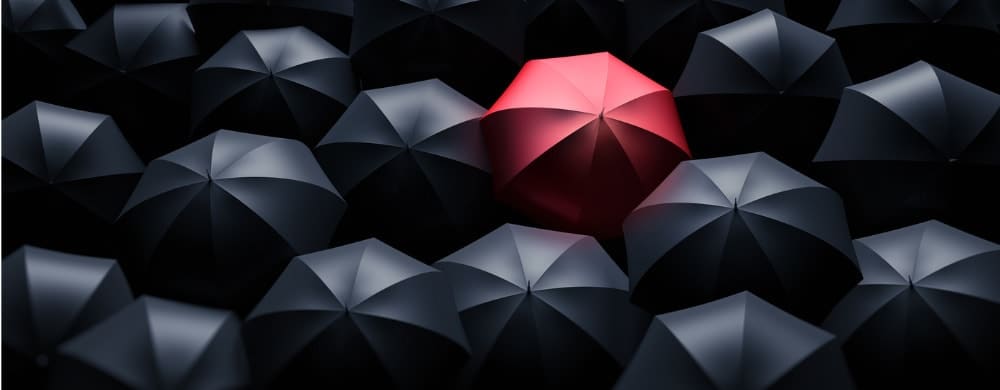
Money Launderers Exploit the Insurance Business
The insurance industry would not appear at first glance to be a fruitful area for money launderers. But it turns out that is not the case. Although the risk is generally viewed as not being of the same magnitude as in other financial industries, the risk is significant. Just to give one example, a Colombian drug cartel, using narcotics proceeds, purchased life insurance policies through various brokers. The policies had cash surrender values, and cartel associates were named as the beneficiaries. These beneficiaries then proceeded to cash out the policies prematurely despite the substantial penalties involved.
The Insurance Industry’s Decentralized Nature Leaves It Open to Money Launderers
Another factor that makes the insurance industry attractive to money launderers is the decentralized nature of its operations. Many policies are sold by independent agents or brokers, who may not be aware of the need for vetting clients. (Occasionally they may even be complicit in the criminal activity.)
What Insurance Companies Need to Watch Out for
To prevent being exploited by money launderers, there are some red flags that insurance companies need to watch out for in issuing policies. Some of them include:
- Purchase of a property insurance policy, followed by a rapid claim
- The purchaser seems more interested in the cancellation terms than the policy benefits or how well the policy performs as an investment
- Making unusually large payments using cash, money orders, or checks.
Insurance Companies Are Subject to Compliance Obligations
The Federal Government has already recognized that the insurance industry is exposed to money laundering activity. Like financial institutions, insurance companies are subject to suspicious activity reporting (SAR) requirements. When warranted, a SAR is required to be submitted to the Department of Treasury’s Financial Crimes Enforcement Network.
Insurance companies also need to run all customers’ identifying data against AML sanctions lists such as OFAC. Matching up two data records, however, can be difficult. Luckily, there’s a technology that can help, Fuzzy Name Matching (also known as Entity Matching).
Why Fuzzy Name Matching is Critical
A data record for a customer will contain standard items such as Name, DoB, PoB, Address, etc. Person names can be especially challenging to match. Here’s a sampling of name phenomena that have to be handled:
- Transliteration variations: Abdel Fattah el-Sisi – Abdul Fatah al-Sisi
- Nicknames in place of given names: Robert vs. Bob, Katherine vs Katie
- Use (or the absence) of middle names or initials : John Frederick Stone vs. John F. Stone vs. John Stone
- Also, due to the great ethnic diversity in the U.S. and increasingly globally, there will be names of many different ethnic types which have their own unique variations. Here are just a few of them:
- Spanish names frequently contain both matronymics and patronymics (surnames from the mother and father, respectively); alternatively, one of them (matronymics) may be dropped: Juan Ramos vs. Juan Ramos Ramirez.
- Foreign languages in Latin script also frequently contain diacritics, which may be absent: Joaquín Guzmán – Joaquin Guzman
- Arabic names, which commonly contain an element “al-,” may appear with or without it, as in Musa al-Rashid vs. Musa Rashid
- Some East Asian names are in the last name + first name order while others are not: Xi Jinping, Moon Jae-in, but Shinzo Abe
In addition to names, other record fields pose their own challenges,
- DoB: 10/9/1992 vs. October 9, 1992
- PoB: UK vs. Great Britain
- Address: Ten Hollifield Lane, Denver, Colorado vs. 10 Hollifield Ln., Denver, CO
Intelligent fuzzy name matching is required to handle all these phenomena.
How Fuzzy Name Matching Works
Traditional fuzzy name matching uses combinations of techniques such as Soundex, Metaphone, Edit Distance, and variations of these. More recently, advanced name matching utilizes Machine Learning to train on extensive real-world data consisting of name variants and generate name matching models. These models not only capture phonetic and string-based variants but other challenging phenomena that were described above. After similarity scores of each record field (i.e., Name, DoB, PoB, Address, etc.) are determined, the results are combined into an overall score for the whole record. Business logic dictates what the weights of each field should be for something to be considered a good match. For example, the weights of the Name and DoB fields may be set higher than those of Address and Phone Number fields because the latter could change during a person’s life time.
In sum, Fuzzy Name Matching is a key technology for the insurance industry in combatting money laundering.