Contact us and see what NetOwl can do for you!
Sentiment Analysis Provides Large-Scale, Real-Time Brand Monitoring
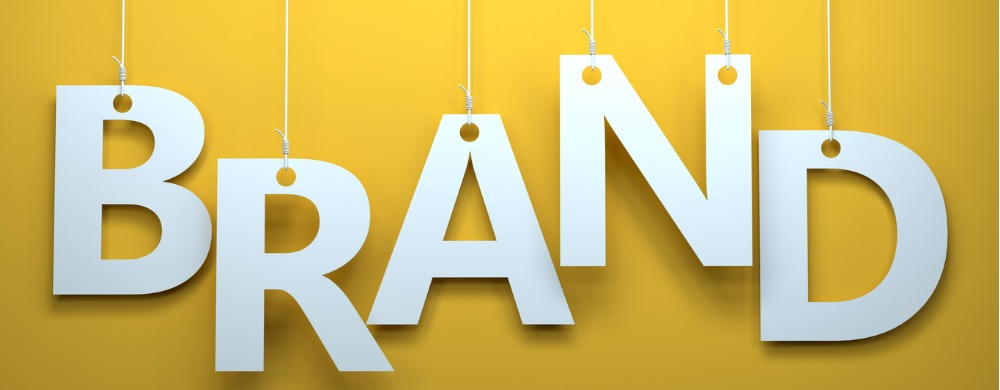
A Business’ Brand is a Precious Asset that is Critical to its Success
Millions of consumers go online every day in order to express their opinions about a company—particularly on social media such as X (formerly Twitter), Instagram, and Facebook—or write reviews of a company’s products and services.
Consumers express themselves on a company from any number of angles:
-
- Quality of its products
- Its customer service
- Its leadership and their actions such as political stance and pronouncements
- Product recalls
- Accidents involving its products
- Effectiveness of its advertisements
- Its treatment of employees as reflected in wages, benefits, hours, promotion opportunities, working conditions, and layoffs
- Its attitude towards union organizing efforts
- Its political involvements
- Scandals, whether financial or otherwise
- etc.
This data is an unprecedented trove of information for companies and can give them an excellent view as to how they are performing in the eyes of customers—about what’s working and what’s not working—and act accordingly.
Sentiment Analysis Empowers a Company to Monitor Its Brand Effectively
It’s essential for companies to monitor their online presence and to be able to do so in real time. Social media posts containing negative information about a company can quickly go viral and be seen by potentially millions. However, monitoring what’s being said about a business is challenging at such a large scale.
Some of the most important information is contained in unstructured text data, which is just ordinary language produced by people or increasingly by AI. This unstructured text data containing customer views is found in many places online, including:
-
- Social media
- Forums, such as Reddit and Quora
- Third-party review sites and review aggregators
- Traditional and non-traditional news sources such as newspapers, blogs, and podcasts.
In the past, businesses have used human teams to study people’s online attitudes towards their brand, but current volumes of data make a purely human solution insufficient and require an automated solution. Luckily, there is an AI technology that can help: Sentiment Analysis.
How Sentiment Analysis Works
Using AI techniques, Sentiment Analysis identifies people’s attitudes in unstructured text data.
Simplistic versions of Sentiment Analysis as offered by some vendors can identify positive or negative sentiments in a text or a sentence by looking for sentiment-bearing words such as “excellent” or “awful.” They do not, however, capture what the sentiment is specifically targeting because they do not understand the context in which those words occur. It is incapable, for example, of handling a post such as the following:
-
- “XYZ Corp’s latest phone upgrade is good, but their tech support is terrible.”
Here two sentiments are being expressed – a positive one towards the phone upgrade and a negative one towards the tech support.
What is needed is a technology that can recognize that two sentiments are being expressed in the same post and can associate them accurately with their respective targets.
A more advanced version of Sentiment Analysis, Entity-Based Sentiment Analysis, achieves these goals. It is able to identify not only sentiments but also the target of those sentiments and any associated entities.
Advanced Entity-Based Sentiment Analysis also makes possible the aggregation of sentiment found in social media posts. This is because it structures and normalizes all the sentiments and entities it identifies. The following examples show typical structured outputs of Advanced Entity-Based Sentiment Analysis:
-
- “XYZ Corp.’s recent product advertisement is deceptive.”
- Sentiment Type: Negative
- Sentiment predicate: deceptive
- Target: product advertisement
- Associated entity: XYZ Corp.
- “XYZ Corp. is the most dishonest company around.”
- Sentiment Type: Negative
- Sentiment predicate: most dishonest company
- Target: XYZ Corp.
- “ABC Corp.’s customer support is truly excellent.”
- Sentiment Type: Positive
- Sentiment predicate: excellent
- Target: customer support
- Associated entity: ABC Corp.
- “ABC Corp.’s new website is a big improvement.”
- Sentiment Type: Positive
- Sentiment predicate: big improvement
- Target: new website
- Associated entity: ABC Corp.
- “PQR Corp.’s new product is really outstanding.”
- Sentiment Type: Positive
- Sentiment predicate: outstanding
- Target: new product
- Associated Entity: PQR Corp.
- “XYZ Corp.’s recent product advertisement is deceptive.”
In these examples, the sentiment type, Positive or Negative, is always identified. In addition, the component elements of the sentiment—the sentiment predicate, the target, and the associated entity—are identified.
This structuring allows sentiments to be aggregated by the sentiment type, predicate, and/or target, typically in a dashboard-style view, and provides companies with a statistically grounded view of its brand reputation. The time stamps associated with social media posts can also give companies a view of how public attitudes are trending over time.
For example, by aggregating over Sentiment Type, a company can see the ratio of positive to negative sentiments as a whole and see how that ratio is trending as well as detect any sudden spikes in either positive or negative sentiment. Similarly, by aggregating over Sentiment Target, a company can see what aspects people are expressing sentiment about, for instance its new product release may be getting a very positive reception, but its CEO is decidedly not.
Summary
Sentiment Analysis is an advanced AI technology for monitoring a company’s brand reputation. It provides companies with real-time access to what the public is thinking about them.
It offers the ability to quickly identify new sentiment trends and monitor ongoing sentiment at scale, making it possible to address issues before they go viral or perform damage control in a timely manner.