Contact us and see what NetOwl can do for you!
Entity Extraction Automates the Redaction of Employee Surveys
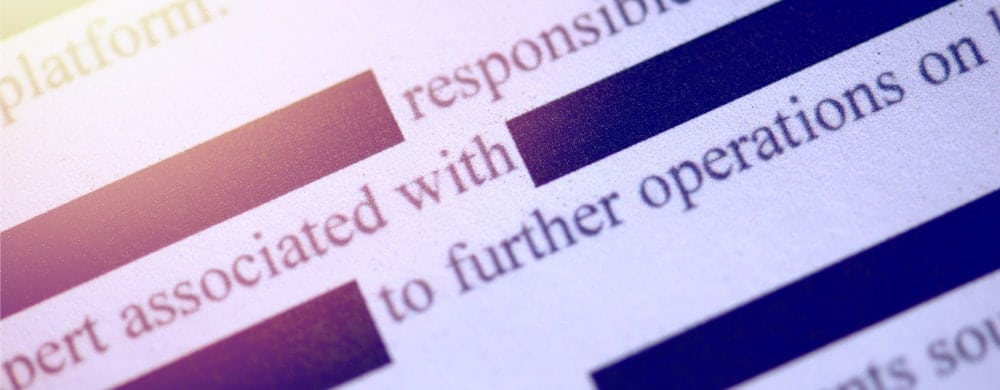
Employee Surveys Are a Critical Resource for a Happier Workplace
Employee surveys are a useful tool in an organization’s toolbox for gaining greater understanding of employees’ attitudes towards all aspects of a business. Traditionally they are a principal means for employees to express their opinions on company policies regarding:
- Pay and benefits
- Quality of management
- Opportunities for advancement
- and many other issues.
Of course, surveys are also used to analyze employee morale and the workplace environment. They may even broach issues of misconduct, whether due to sexual harassment in this age of #MeToo or to more general issues of managerial abuse of employees, favoritism, or anything that comes under the rubric of a hostile work environment.
Companies Need to Protect Sensitive Data in Employee Surveys
Of course, many employees feel that responding to even an anonymous survey will expose them to potential retaliation. For that reason companies frequently bring in a third party to administer the surveys and analyze the results.
A critical step in this process is redaction (also known as data masking). Redaction is about hiding or removing sensitive data that might reveal the identity of an individual. It’s easy enough to simply hide the structured data in a survey (items in their own data fields such as title, location, etc.). It’s more difficult, however, to handle the unstructured data, such as comments that employees might make in ordinary language in the free-text fields that the survey contains. These comments might advertently or inadvertently include sensitive information that should be confidential. There’s a technology, Entity Extraction, aka Named Entity Extraction and Named Entity Recognition, that can help eliminate that danger.
How Named Entity Extraction Keeps Sensitive Information Confidential
First and foremost, all personal names occurring in the unstructured portions of a survey have to be identified, which is the first step to redacting them. This used to be, and still can be, done manually by humans, but many third-party organizations that conduct surveys on behalf of other organizations have discovered that’s not a good use of their time since it is quite time-consuming. Entity Extraction does it automatically. For example, consider the following example:
- “John Jones frequently dresses down employees in public in a very abusive way”
Entity Extraction identifies that “John Jones” is a person name. It doesn’t do this by maintaining a list of all possible names. This is, in fact, impossible, since the number of possible names is indefinitely large: the great majority of possible first names is indeed fixed for the most part (though new first names are always being created – think “River” in “River Phoenix”), but the set of possible last names is pretty open.
How Entity Extraction Works
Entity Extraction uses linguistic information as well as contextual clues such as titles or honorifics surrounding the personal name to identify words as a name and to establish its type; in this case “John Jones” will be classified as a Person Name. This allows Entity Extraction to identify names dynamically, i.e., it can recognize person names it has not encountered before.
Entity Extraction also identifies other entity types that may occur in survey comments, for example,
- Organization names
- Telephone numbers
- Email addresses
- Office locations
In addition, expressions like “Accounts Receivable” may appear that could provide a clue to where in the organization the commenter resides, such as in the context “I work in Accounts Receivable.” Again, Entity Extraction recognizes “Accounts Receivable” as an organizational unit and allows it to be redacted.
A more advanced capability of Entity Extraction is co-reference resolution. Personal names frequently appear upon first mention in their complete form, “John Jones,” but then in a shorter form in any subsequent mentions: “John” or “Jones.” Co-reference resolution is the capability of identifying “John” or “Jones” as an alternate form of “John Jones” within a text so all these instances can be identified as the same person. Then co-reference resolution can replace these instances with the same variable PERSON1, and so maintain the complete context for understanding the content of the survey response. Likewise, if two or more entities of the same type are mentioned in a single comment, it is necessary to differentiate them, e.g., “PERSON1” and “PERSON2,” as this also maintains the accurate meaning of the comment.
In sum, Entity Extraction provides a unique capability to find and extract the sensitive information within unstructured text. It automates a process that had previously been a tedious and time-consuming effort that an organization would have to carry out entirely by hand.