Contact us and see what NetOwl can do for you!
How Sentiment Analysis is Critical for Successful Vaccination Campaigns
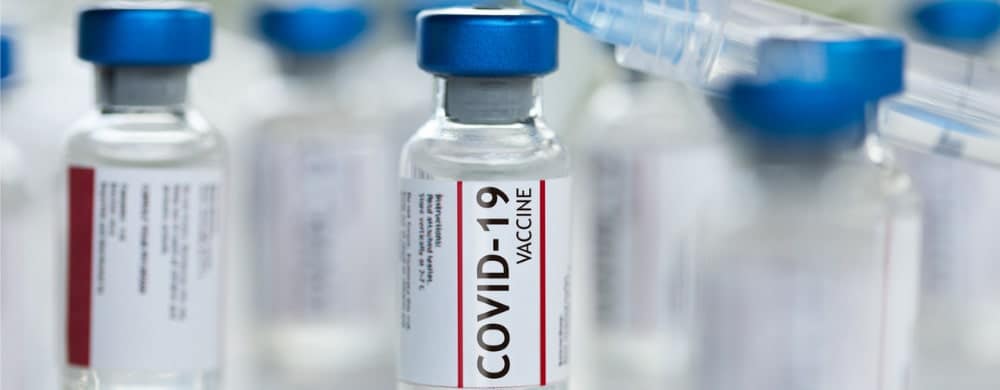
Public Confidence in Vaccines
Immunization is clearly among the greatest of public health achievements. Despite these successes, though, recent years have seen increasing difficulty in maintaining rates of vaccinations that are high enough to reach herd immunity. For one thing, many people believe that the risks of vaccines in general outweigh their benefits. In addition, some recent disease outbreaks have been due to increased public resistance to the MMR vaccine in particular, sometimes due to anti-vax activists and sometimes for religious reasons.
Especially with the very recent development of a safe and effective COVID-19 vaccine, public health authorities need a strategy for maximizing public acceptance of the vaccine. There’s been a fair amount of concern that the development of this vaccine was too rushed and subject to political pressures. It’s critical that public health authorities be able to effectively counter these attitudes. The first step, however, is to increase understanding of precisely what the public is concerned about.
Social Media Provides a Great Deal of Data on Public Attitudes towards Vaccinations
Fortunately, there’s a good source for data on public attitudes towards the COVID-19 vaccine: social media. It offers a wealth of individual attitudes on all aspects of the vaccine, such as contained in the following tweet: “I just don’t trust these politicians pushing this vaccine”
However, social media presents a challenge, since the most valuable information it provides is contained, not in the metadata, but in ordinary human language as in the example above. It has not been easy to analyze it automatically with high accuracy and at the great scale required. However, more recent advances in Sentiment Analysis technology can assist automated analysis of public attitudes toward vaccines in a more effective way.
How Sentiment Analysis Works
Sentiment Analysis uncovers the attitudes expressed by people in unstructured natural language.
It identifies opinions and intentions by using techniques derived from AI and Machine Learning. It performs text analytics of the contents of these sources, which are all unstructured text data, looking for specific sentiments. It finds these sentiments and then outputs them in a structured format.
Sentiment Analysis is also able to process at scale, easily handling very large amounts of data, thus exceeding by many orders of magnitude the capabilities of purely human-driven monitoring.
In the simplest approach, positive phrases like “great” or negative ones like “terrible” are used to indicate that the entire sentence or text is to be classified as positive or negative sentiment. A critical later advance is the ability to connect a sentiment expressed in text to a specific item in the context, i.e., feelings concerning vaccines, public health officials, pharmaceutical companies, or really anything at all. This more advanced version of the technology is called Entity-Based Sentiment Analysis.
Here are examples of how Entity-Based Sentiment Analysis produces a structured representation of the meaning of the sentences below:
- “covid vaccine side effects are really bad like shortness of breath, severe headaches, irritation to the skin, insomnia and lots more.”
- sentiment:negative
- entity: covid vaccine side effects
- “big pharma is bad news they’re pushing an unproven vaccine to reap huge profits” (two sentiments expressed)
- sentiment:negative
- entity: big pharma
and
- sentiment:negative
- entity: an unproven vaccine
- “I trust dr fauci I’ll follow his recommendation on the vaccine”
- sentiment:positive
- entity: fauci
- “operation warp speed really did a great job”
- sentiment:positive
- entity:operation warp speed
In the first two examples, the sentiment expressed is negative, in the last two positive.
Each sentiment is also linked to objects of sentiments (entities) that the public expresses opinions about. The examples here are “covid vaccine side effects,” “big pharma,” “an unproven vaccine,” “dr fauci,” and “operation warp speed.”
Obtaining information on WHAT specifically a commenter is happy or unhappy about gives researchers good insight into what the public’s exact concerns are about the vaccine.
The fact that the output of Sentiment Analysis is structured, i.e., it has a predictable format that has eliminated all the ambiguity of natural language, means that it is easy to aggregate and track trends over time.
How Sentiment Analysis Can Be Used to Understand Public Attitudes towards Vaccination
The insights derived from Sentiment Analysis provide a clear basis for designing a public health campaign to increase support for vaccination by taking into account the public’s concerns and fears. Since the data is structured, researchers can study trends over time in the nature of the sentiment. For example, it will now be possible to track issues such as the following:
- Is willingness to be vaccinated going up or down?
- What are the top reasons for the public’s hesitancy about getting vaccinated?
- What motivates the public the most to get vaccines?
The results of Sentiment Analysis can also be combined with the demographic data to study how sentiment varies among different populations. This would aid in developing effectively targeted campaigns.
Summary
In sum, Sentiment Analysis is a valuable tool that will give public health researchers and officials fine-grained information on the issues surrounding the COVID-19 vaccine that concern the public.
It will enable them to craft an empirically-based public health campaign to maximize public acceptance of the COVID-19 vaccine.