Contact us and see what NetOwl can do for you!
Is Your Customer Credit Worthy? NetOwl Helps You Figure It Out
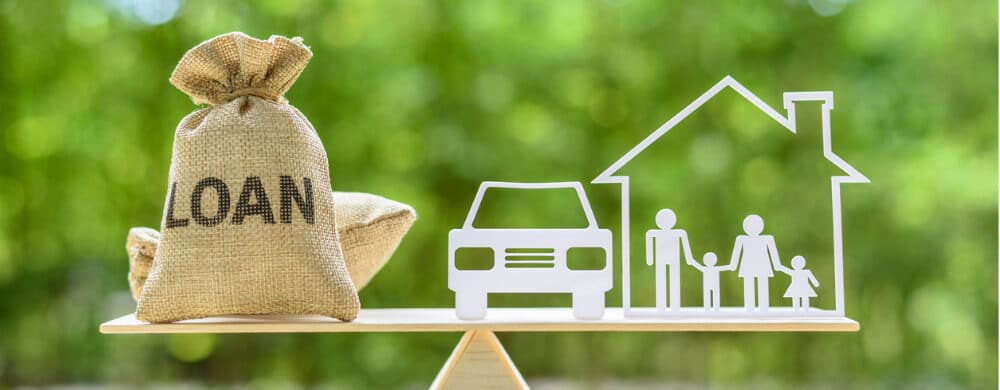
Lenders Need to Know the Credit Worthiness of Their Customers
Lenders nowadays span a wide range of companies, from traditional credit card companies and banks to retailers and digital payment services such as PayPal, Venmo, and Apple Wallet. Whether for a loan, line of credit, or credit card, lenders need to perform credit checks of applicants.
Lenders typically use a third-party credit-checking service such as Experian or Equifax to match an applicant’s data against known bad actors. But some lenders also have their internal audits to monitor the accuracy of these matches and to make sure that they are following due process (e.g., for KYC and AML). As part of those audits, lenders may need to perform their own evaluation on their matched data. To do this, they typically establish a test set of applicant data containing both the applicants’ personal data (name, date of birth, etc.) and the matches returned by the third-party service. This evaluation is a labor-intensive process, as lenders make every effort to determine the actual accuracy of a match using other credit-checking services and any other data they can find.
Identity Resolution is an AI-based technology that can support this evaluation process. It allows the lender to monitor the accuracy of what the third-party credit-checking service is returning.
Matching Data Fields Is a Challenging Problem
There are complex challenges involved in matching data fields containing credit application data. For example, here are some phenomena commonly found:
- Use of different forms of a name such as a nickname: Robert vs. Bob
- Simple typos
- Spelling variants: Sean vs. Shawn
- Ethnicity-specific phenomena:
- Hispanic personal names typically contain a patronymic and matronymic. The latter is frequently dropped: Hector Garcia-Lopez vs. Hector Garcia.
- Arabic names frequently contain the definite article “al.” It is frequently left out: Haroun al-Rashid vs. Haroun Rashid
- Women still frequently change their surname after marriage.
- Addresses exhibit frequent variations:
- Numerical vs. written notation: 12 Maple St vs. Twelve Maple St
- Abbreviations: Avenue vs. Ave, West vs. W.
- Varying order of elements (“Paris 75008” vs. “75008 Paris”)
- Translated elements (“Wien, Österreich” vs. “Vienna, Austria”)
- Dates have multiple possibilities: 10/09/72 vs. 10/09/1972 vs. October 9, 1972 vs. Oct. 9, 1972.
Identity Resolution Is a Technology That Can Help
In order to determine the accuracy of the third-party data, what is needed is a technology that can match on each data field individually and return a score indicating how close the match is. For each field, it needs to apply matching that is suitable to the characteristics of that field.
The technology also must be able to return a combined score for all fields. It needs to be highly accurate, minimizing both false positives and false negatives.
Both the individual field scores and the combined ones will provide the lender with a fine-grained view of how good a match the data returned by the third party really is. This will also contribute to the lender’s being better able to meet its KYC and AML requirements.
How Identity Resolution Works
Advanced Identity Resolution is a technology that meets the requirements above. First, it matches in a fuzzy way, using matching models derived from Machine Learning algorithms that are trained on a large amount of real-world data. It scores matches on each field (e.g., person name, address, DoB) to indicate degree of similarity and also provides a combined score for all fields. Finally, it allows user to create business rules that determine the threshold for a good match.
Summary
It’s critical that a lender has highly accurate matching of credit applicants. Identity Resolution can play a valuable role in assessing accuracy by providing specific, quantitative assessment of how close matches are at both the individual field level and for the combined fields.