Contact us and see what NetOwl can do for you!
How Entity Extraction Aids Business Intelligence
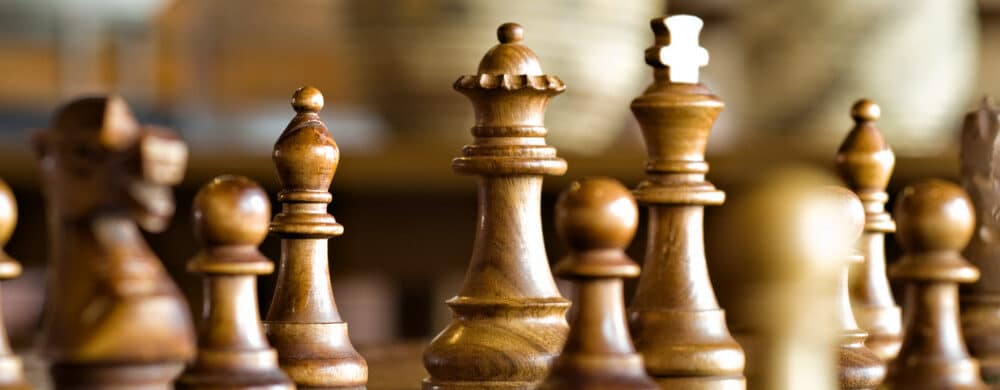
Good Competitive Intelligence Is Critical for a Firm
Business intelligence is required for a firm to stay competitive. It needs to know how the industry is reshaping itself to meet new imperatives emerging from technology advances, government policies, and all the other factors that might affect it.
Firms Need to Monitor Their Industry
For that reason, firms need robust ways of monitoring developments in their industry such as:
- What acquisitions or mergers or partnerships their competitors might be mulling over
- Personnel changes at the C-level.
- What new sectors they are planning to move into
- What new products they are planning to launch
Corporations need this information in order to form effective strategies for competing.
Unstructured Text Data Is an Important Source of Information
Probably the earliest indicators of changes and new developments in an industry are contained in unstructured data such as news and social media. Another source of critical data is that of public filings by corporations to the SEC. Unstructured data, however, is also difficult to mine. For one thing, its volume is immense. Natural language is rich and exhibits a great deal of variation, both syntactic and lexical, which results in many potential ways of conveying information. Critical information may be contained in foreign language media.
Fortunately, there’s a technology that can help: Entity Extraction.
Entity Extraction for Business Intelligence
Entity Extraction is an AI-based technology that identifies key concepts in unstructured text. At its most basic level, it identifies names of people, organizations, places, numerical amounts (money, etc.), dates, and times. (This is called Named Entity Recognition or Extraction.)
Advanced Entity Extraction identifies more sophisticated concepts. It can analyze, for example, news reports on a company’s personnel changes. Entity Extraction will identify the names of all executives incoming and outgoing. It will identify the positions they are entering and leaving. Consider the following example from, say, a business magazine:
- XYZ Corporation announced that its long-time CFO Jonathan Miller will step down effective April 1st. He will be replaced by Meredith Barnes.
Entity Extraction will process this and produce structured output like:
- PERSONNEL_CHANGE_EVENT
- CORPORATION: XYZ Corporation
- POSITION: Chief Financial Officer
- OUTGOING_EXECUTIVE: Jonathan Miller
- INCOMING_EXECUTIVE: Meredith Barnes
In order to produce this output, Entity Extraction first recognizes the names of the corporation and the two individuals as well as other references such as pronouns and common noun phrases. Entity Extraction does not rely on long lists of known names to do this. It uses the context to recognize names and what kind of entities they represent. This is usually termed dynamic recognition, and it is one of the great strengths of Entity Extraction.
Entity Extraction then recognizes the relationships between individuals and companies as well as the relevant events, such as a personnel change event, that a company is involved in.
There are many other events and relationships of interest from a competitive intelligence point of view. For instance:
- Product recalls (e.g., “Ford issues three major safety recalls with 40,000 owners affected”)
- Mergers and acquisitions (e.g., “Wisconsin Energy and Northern States Power agreed to a $6 billion stock merger that would create one of the 10 biggest investor-owned utilities in the nation”)
- Making a bid on a business (e.g., “The company said Friday it and its consortium partners had made a counter-bid for Club Méditerranée SA”)
There have been efforts in the past (and currently on-going even) to use a team of humans to track this kind of information. This human-only approach has severe maintainability issues, as it’s very difficult to keep up with new firm formation. An approach like Entity Extraction that can recognize previously unseen names and other concepts reliably is far superior.
Entity Extraction is also fast, so it can produce very large amounts of structured data very quickly. Since it’s structured, it can easily be stored in a database. It will be searchable just like data that was structured to begin with. It can also be aggregated – an event label like PERSONNEL_CHANGE_EVENT will not vary, so it’s easy to develop a statistical view of such events over time and across an industry.
In sum, Entity Extraction offers a very attractive and fast means of generating competitive intelligence.