Contact us and see what NetOwl can do for you!
How Relationship Extraction Enhances Link Analysis
Entity Extraction, Homeland Security, Intelligence Analysis, Risk Management
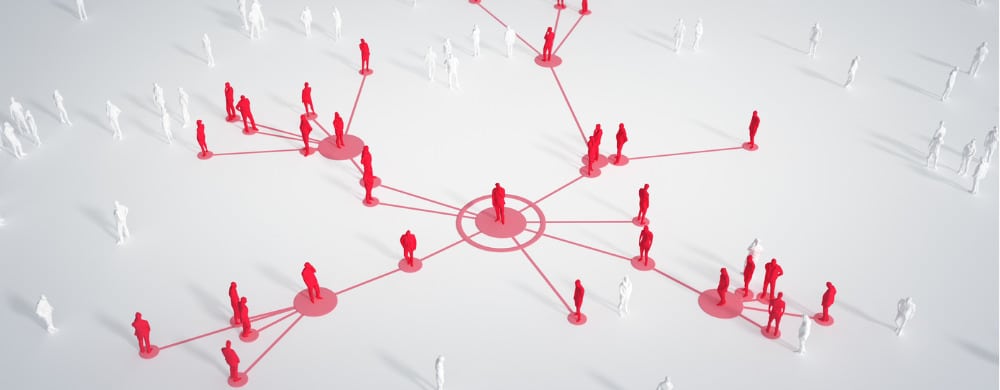
Link Analysis is a Critical Tool for Knowledge Discovery
Link Analysis is a well-known analytical technique used for knowledge discovery. It discovers relationships in data through network visualizations. Typical relationships that are of interest in many domains include those between:
- Person and organization (e.g., employment)
- Person and person (e.g., parent)
- Organization and organization (e.g., subsidiary)
- Person and place (e.g., place of residence)
- etc.
Link Analysis supports a “six degrees of separation”-style investigation where the search can be extended out through multiple nodes in the network.
Since the network visualization offered by Link Analysis is so helpful to humans in discovering significant and otherwise hidden links in data, it is used extensively in many different domains such as the following:
- Due Diligence for Risk Management
Financial services firms have Know Your Customer (KYC) requirements for potential clients. Link analysis enables them to explore the organizations that the client under review has been associated with as well as the individual’s business associates and any other connections.
- Fraud Detection
All industries have to guard themselves against fraud. Some of the most exposed to it include financial firms, credit card issuers, and insurance companies. The federal government has to watch out for Medicare/Medicaid and tax fraud. Link analysis is a fundamental tool in the battle. It offers organizations a natural and intuitive way of investigating patterns of association that may indicate the presence of fraud.
- Law Enforcement
Law enforcement personnel frequently use Link Analysis techniques and tools in investigations into criminal activity. For example, officers can investigate the structure of organized crime groups and the relationships among their members and discover otherwise hidden associations.
- National Security
Intelligence analysts use Link Analysis tools in the investigation of adversaries, such as terrorist networks and cyber hackers. It allows them to study the networks that these actors are embedded in. For example, visualizing the travel activities of members of a group (i.e., links between individuals and locations) is a critical piece of knowledge for evaluating their operational intentions.
The Huge Quantities of Unstructured Data Now Available Require Automated Extraction to Support Link Analysis
In all of the domains mentioned above, a critical source of relevant data in many areas is unstructured text data. Traditionally, users would read material from unstructured text containing information on relationships and then manually build link diagrams reflecting that information. This is a slow and cumbersome approach that does not scale, and it is not a good use of the analyst’s precious time.
In addition, the recent huge increases in the availability of unstructured text, as for example on social media, has made it pretty much impossible to stay on top of data using exclusively manual means no matter how big the team is.
What is needed is a technology that helps the analyst both collect relevant information from huge quantities of unstructured data and uncover relationships that are critical for a successful analysis.
Luckily there is a technology that can help: Relationship Extraction. It automates the identification of critical links between entities in unstructured text. It allows an analyst to engage in real knowledge discovery, i.e., an analyst can devote their attention to identifying the key information and finding patterns, and not to the reading of voluminous unstructured text. The task of finding relationships has been automated by Relationship Extraction.
How Relationship Extraction Supports Link Analysis
Relationship Extraction doesn’t just identify co-occurrences of entities in unstructured text. It identifies the precise semantic relationship between them. It then outputs a structured representation of the relationship, which can be stored in some other kind of database. The structured relationship data can then be exploited by a Link Analysis tool.
We give three examples below of three different types of relationships that Relationship Extraction identifies. For each example we provide the unstructured text that Relationship Extraction processes and then the structured representation of the relationship that it outputs.
- An employment relationship between a person and an organization
Audrey Crossman is regional vice president of sales for Celera Inc.
Relationship Type: Affiliation
Person: Audrey Crossman
Organization: Celera Inc.
- Family relations
Slobodan Markovic’s wife Mirjana Mladic
Relationship Type: Spouse
Person: Slobodan Markovic
Person: Mirjana Mladic
- Business associates
Former boston cabdriver Nabil Almarabh, who has ties to Rajad Khalid
Relationship Type: Associate
Person: Nabil Almarabh
Person: Rajad Khalid
Relationship Extraction uses a semantic ontology for predefined relationships to label the occurrence of a relationship in unstructured text. Natural language can express the same relationship in many different ways, and Relationship Extraction has the major advantage of outputting the same structured representation regardless of linguistic variations. For more information on how Relationship Extraction works, see our other blog here.
The Combination of Relationship Extraction and Link Analysis Greatly Enhances Knowledge Discovery
Relationship Extraction has the capability of processing today’s increasingly staggering quantities of unstructured text data at scale. The output of Relationship Extraction from a large volume of text is available to Link Analysis tools. There is no longer a need for extensive manual input.
For example, a business analyst researching a company can build a detailed organization dossier by automatically analyzing news articles and press releases. Relationship Extraction identifies, for example, executives who are hired or promoted as well as other companies that the company has recently acquired, partnered with, or received investment from.
In analogous fashion, in the intelligence analysis application, Relationship Extraction can be applied to a large volume of available reports and messages to uncover, for example, membership information of a terrorist group as well as relationships among different terrorist organizations.
In another scenario, as part of due diligence, a financial institution may be able to establish that a potential customer or a supplier has a business associate or a relative who was previously charged in a fraud case, thanks to the KYC data that incorporates the latest up-to-date news that is analyzed and structured by Relationship Extraction.
Summary
In all of these scenarios, Relationship Extraction identifies crucial links between entities in unstructured text. The combination of Link Analysis and Relationship Extraction greatly enhances true knowledge discovery far beyond what humans can do on their own and at the same time offer substantial cost savings in producing such knowledge.