Contact us and see what NetOwl can do for you!
Sentiment Analysis Helps to Understand the Public Response to Pharmaceuticals
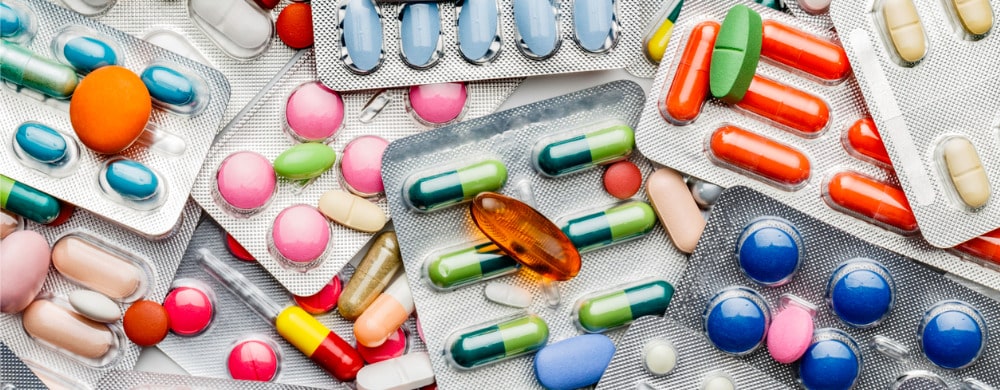
There’s a Ton of Clinical Data on the Internet Expressed in Unstructured Data
Many users of the Internet currently communicate on medical forums and social media (Twitter, Facebook, etc.) in order to gather health-related information. One area of particular interest is pharmaceuticals. People are eager to record their own experiences with drugs, and they also want to hear about what other people are saying. Subjects that receive a lot of attention include:
- Efficacy
- Side effects
- Price
- Comparative value of brand name vs. generics
Many people comment at length. All of this constitutes an enormous amount of clinically relevant data that, when properly analyzed, could produce many insights that would be invaluable. Never before has there been such a large volume of self-narrated data on how people experience pharmaceutical therapies.
One of the main challenges of handling this data is its sheer volume. It’s become impossible to analyze its contents by hand. Luckily, there’s an AI-based technology, Sentiment Analysis, that can analyze comments very quickly at the scale necessary to capture statistically valid insights about people’s attitudes to various aspects of pharmaceuticals.
Another challenge with this data is that it is expressed in unstructured natural language. This means that two patients can express similar sentiments about a drug in completely different ways, using very different vocabulary and syntax. This makes it difficult to aggregate sentiment over a large population. Sentiment Analysis can help here, too.
Sentiment Analysis Provides a Structured Representation of Peoples’ Comments on Drugs
Sentiment Analysis identifies opinions and intentions in text by using techniques derived from natural language processing (NLP). It performs a deep linguistic analysis on the contents of patients’ comments, looking for specific sentiments. It then produces a structured representation of these sentiments.
In the simplest approach to performing Sentiment Analysis, positive words like “great” or negative ones like “terrible” occurring in people’s comments are used to indicate that the entire sentence or text is to be classified as positive or negative.
A more advanced approach offers the ability to connect a sentiment expressed in the comment to a specific item in the context, i.e., feelings concerning particular products, persons, or really anything at all. This more advanced technology is called Entity-Based Sentiment Analysis.
Here are some examples as they would be analyzed by Sentiment Analysis. First some positive :
- “Xarelto works well I haven’t had any of the known side effects”
- sentiment: positive
- entity: Xarelto
- “Clindacymin is awesome – my dentist recommended it. My gums stopped bleeding immediately”
- sentiment: positive
- entity: Clindamycin
- “Pentaprazole worked and didn’t bother me at all. My GERD symptoms are much better J”
- sentiment: positive
- entity: Pentaprazole
Then some negative:
- “Lipitor isn’t improving my cholesterol”
- sentiment: negative
- entity: Lipitor
- “Xarelto is too damn expensive”
- sentiment: negative
- entity: Xarelto
- “Divalproex is making me dizzy”
- sentiment: negative
- entity: Divalproex
In each example, the sentiment expressed is classified as either POSITIVE or NEGATIVE. Each sentiment is also linked to a specific drug.
How Sentiment Analysis Enhances Clinical Data
In the past patients’ comments on drugs were restricted to individual doctor’s offices or hospitals, and in the best case, clinical trials for FDA approval. Information coming from medical forums and social media will deepen understanding of how people are experiencing the drugs they are taking.
In particular, the insights from Sentiment Analysis will allow better understanding of a pharmaceutical’s reception among the population including:
- What do people think of a drug’s efficacy?
- What side effects do they report?
- What is the common reaction to the pricing?
Since the data produced by Sentiment Analysis is structured, researchers will be able to establish a precise, quantitative assessment of each of these factors. In addition, if metadata such as time stamps on the messages (tweets, comments in medical forums, etc.) is available, it will be possible to track trends over time. In this way, researchers can monitor on a real time basis how the drug is performing.
In sum, Sentiment Analysis makes possible the use of an entirely novel and potentially crucially important data source of unstructured data.