Contact us and see what NetOwl can do for you!
How to Choose an Entity Extraction Product
Enterprise Search, Entity Extraction, Homeland Security, Intelligence Analysis, Risk Management, Social Media Analysis
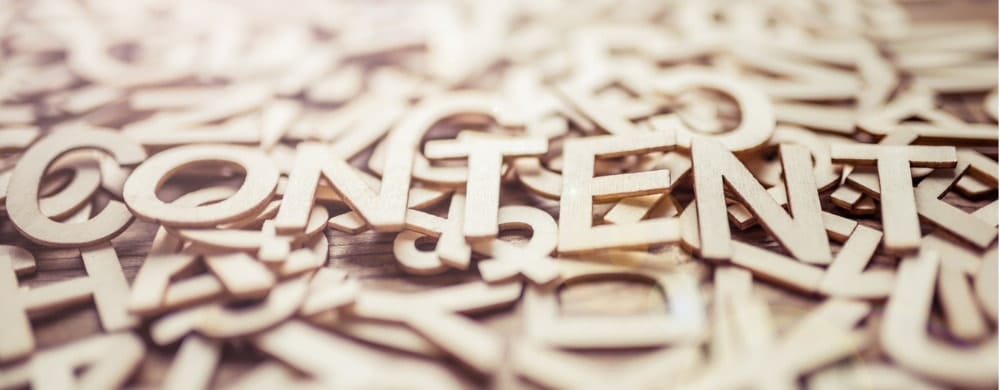
Entity Extraction Is Essential for Organizations to Maximize the Value of Their Unstructured Data
Entity Extraction is advanced AI technology that recognizes key concepts in unstructured text and converts them into semantically structured data. It was pioneered in the nineties through funding primarily from the US Government in order to develop a technology that could make sense of the very large amounts of unstructured data that the Government increasingly had to deal with. As one of the Government managers behind the technology once said:
“We sure knew how to collect all sorts of unstructured data, but we didn’t have any tools for finding the critical things in it and converting them into useful intelligence.”
Entity Extraction Has Gone Mainstream
Fast forward to today and Entity Extraction has escaped the R&D lab and is the focus of a large community of developers. There are a large number of companies offering products. Entity Extraction has matured to the point where Government and business organizations increasingly see a compelling need to include it as part of their digital strategy for realizing maximum value from unstructured data.
Companies that are using Entity Extraction are seeing business impact in many areas, including:
-
- Enterprise search
- Business intelligence
- Intelligence analysis
- E-discovery
- Risk management
- Health care
All Entity Extraction Products Are Not Created Equal
The choice of which extraction vendor to go with is a critical one. There are certain things to keep in mind when mulling over which vendor’s extraction product to choose.
As part of the business case to acquire entity extraction software, an organization needs to consider several factors:
1. Ontology Coverage
The word “ontology” refers to the set of concepts that an entity extraction tool can identify in text. Most extraction tools on the market cover basic “named entity” types, such as:
-
- Person
- Organization
- Place
- Numeric (Money and Percentage)
- Dates and times
One thing to look for is how fine-grained an ontology your application requires. For example, most entity extraction tools will only identify that something is an organization name and not provide any further characterization. They do not distinguish among organizations that might be companies, governmental, educational, non-profits, etc. Similarly some tools extract place names but do not distinguish countries, states, cities, etc. If such distinctions are critical to your planned application, then you want to look elsewhere.
2. Accuracy
Another critical factor is an entity extraction tool’s accuracy. Extraction has an inevitable error rate, and it’s important to find one that minimizes both false positives and false negatives.
A false positive is to extract something as, e.g., a person name that isn’t one. For example, “Douglas firs” could be taken to be a person name because of the apparent first name. It’s not, obviously.
A false negative is simply to miss a name that should have been extracted. Typically a metric called F-Measure is used to measure the accuracy of entity extraction taking into account both false positives and false negatives.
3. Coverage of Different Types of Texts
Related to the previous section, accuracy may actually vary greatly depending on what types of texts are used as input. Ideally you’d like an entity extraction tool to be able to process a wide variety of unstructured text data with high accuracy, from well-edited news reports to all-lowercase email to wildly ungrammatical tweets. If your input texts include not-so-well-edited documents, but your entity extraction tool is hampered by grammatical irregularities or is dependent on correct capitalization of names, then it will likely not meet your requirements.
4. Foreign Language Coverage
Do you need extraction from texts in foreign languages in addition to English? This is important to know since entity extraction tools differ widely in their language coverage.
5. Scalability
If the amount of your text input is not trivial, you want to ask if an entity extraction tool can handle the volume of unstructured data that needs to be analyzed within your time frame. In addition, given the constant increase of unstructured data on Social Media, the Web, as well as other sources, you may want an entity extraction tool to be highly scalable. In other words, could it be easily deployed to more servers to handle any future increases?
6. Customizability
For named entities, the range of desirable extraction targets can range from the ordinary such as person and organization names to the very arcane or technical such as those found in scientific or technical domains (e.g., widget names in a specialized area). No entity extraction tool on the market covers all the bases. An organization needs to determine the following:
-
- Can the entity extraction tool extract what is needed out-of-the-box?
- If not, can the extraction tool be customized to handle what is needed?
- How easy and quick is it to customize?
7. Advanced Extraction Capabilities
Few entity extraction tools have the ability to go beyond extracting basic named entities and extract more advanced semantic concepts such as relationships and events. A relationship is a semantic association between two entities, such as an affiliation relationship between a person and an organization or a familial relationship between two people.
An event is more complex, generally involving an activity in which entities participate along with a date and location of the event. For example, for a sentence “Salesforce acquired Tableau in 2019,” event extraction would extract an Acquisition event with two participants, the acquirer (Salesforce) and the acquired company (Tableau), and the purchase date (2019).
Some applications may only require named entities. For example, you are planning to develop a faceted search application where named entities are used as search filters. However, other applications might need advanced extraction. For instance, you need to monitor a large amount of news and other text data real time in order to discover any adverse events (e.g., arrest, lawsuit, indictment) and perform due diligence. An organization must think carefully about whether it needs basic or more advanced extraction.
Summary
In sum, we have listed a variety of factors a potential user of entity extraction may want to consider before making a decision. The technology is no longer just a “niche” product, and it is been used in many large-scale production environments. The bottom line, though, is that users need a good understanding of their requirements and the capabilities of each extraction product in order to make the right choice.