Contact us and see what NetOwl can do for you!
How to Choose a Sentiment Analysis Product
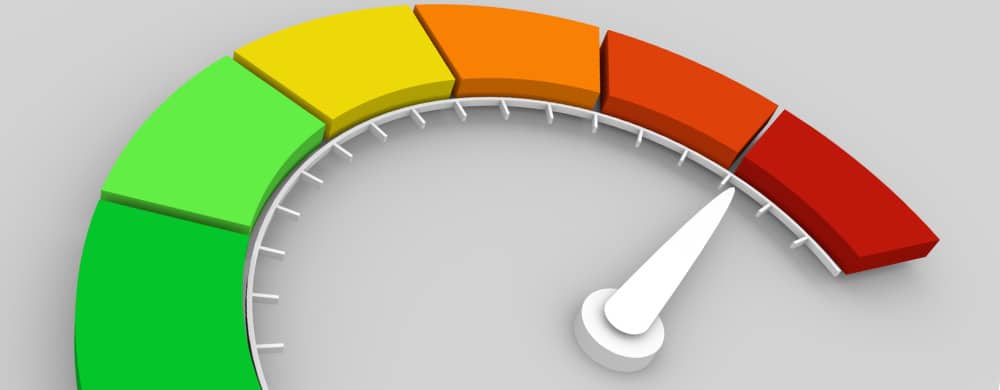
What is Sentiment Analysis?
Sentiment Analysis is a technology that identifies the sentiments expressed in unstructured natural language as found in sources like email, tweets, or comment pages. The sentiments may be expressed towards many things, such as:
- People
- Places
- Organizations
- Products
- Movies
- and just about anything else.
In particular, companies have an increasing need to make use of sentiments expressed towards them. Items for which they need to monitor public reactions include:
- Quality of products and services
- Pricing
- Social consciousness or lack of it
- etc.
How Is Sentiment Analysis Used?
Sentiment Analysis has many uses in different industry sectors. Here are a few examples:
- Public Opinion Polling
- A news organizations wants to survey public opinion on an issue.
- Market research
- A company is planning a new product and needs to understand what features it should have to succeed in the marketplace.
- Brand/Reputation monitoring
- A company needs to monitor how the company and its product(s) are perceived, particularly on social media.
This blog won’t discuss how Sentiment Analysis works. For that see one of our other blogs.
This blog is devoted to the criteria for choosing a Sentiment Analysis Product.
How to Choose a Sentiment Analysis Product – What Are the Criteria?
1. Does it handle sentiment at document-level or entity-level?
Sentiment Analysis tools on the market today come in different flavors.
- The simplest ones look for positive phrases like “great” or negative ones like “terrible” in the entire text or individual sentences in order to characterize the sentiment as Positive or Negative.
- A more sophisticated kind of tool includes the ability to connect a sentiment expressed in text to a specific item in the context, i.e., feelings concerning particular products, persons, or really anything at all. This more advanced technology is called Entity-Based Sentiment Analysis.
This type of Sentiment Analysis goes deeper and analyzes the sentiments expressed about specific features of the entities, providing a company with a rich set of data to analyze. For example:
-
- “The Hotel Eventi was amazing. Its location was excellent, the room was spotless. One thing I did find fault with is the price.”
- Here there are four distinct sentiments expressed:
- Overall reaction to the hotel (“amazing”)
- Location (“excellent”)
- Room (“spotless”)
- Pricing (“I did find fault with”).
An organization’s use case would dictate which types of sentiment analysis is more useful to them.
2. Does it have a rich sentiment ontology?
A sentiment ontology is the organized list of different kinds of sentiments captured by a product beyond positive and negative. Each sentiment is assigned to a particular sentiment type within the ontology. Some examples include:
- Positive Sentiment
- RECOMMEND:
- “You should buy your beer at Costco”
- INTEND TO BUY:
- “I’m buying an electric car.”
- Negative Sentiment
- CALL FOR BOYCOTT
- “Let’s boycott XYZ’ products!”
- CRY FOR HELP:
- “I need help with my ipad”
- CALL FOR BOYCOTT
- RECOMMEND:
The significance of the ontology is that it allows sentiments to be very fine-grained. A tweet is not just labeled POSITIVE or NEGATIVE, but the precise nature of the sentiment is identified, i.e., whether something is being recommended, complained about, etc.
3. Does it allow intelligent aggregation of the sentiment?
If entity-based sentiment analysis is selected, you want to make sure the target entities of each sentiment are normalized linguistically so that big data analysis over the output of entity-based sentiment analysis is much more useful. For example, suppose reviews of a training course contain thousands of positive comments like below:
- “The instructors were clear.”
- “Our lead instructor was easy to understand”
- “I really enjoyed how my instructor interacted with the participants”
- …
By capturing that the normalized entity “instructor” is the sentiment object of these thousands of positive sentiments, one can conclude quantitatively that the instructors did a great job in this training course.
4. Does it handle many different types of texts?
The Sentiment Analysis products needs to have been trained and tested over many different text types:
- Tweets
- Blogs
- News
- Customer surveys
- etc.
The grammar and vocabulary used in each of these sources is quite distinctive, and the product needs to handle the syntactic and semantic quirks found in them.
5. Does it support multiple languages?
An international product firm needs to know how its products are being perceived in many countries. A Sentiment Analysis product needs to provide extensive multilingual coverage.
Summary
As we showed above, the Sentiment Analysis products available in the marketplace today have different capabilities. It’s important for an organization to use the right selection criteria in evaluating products. The criteria we have provided above should be helpful to any business that is considering deploying Sentiment Analysis. Using them will lead to a choice that is the most effective for your requirements.