Contact us and see what NetOwl can do for you!
How the Combination of Entity Extraction and Name Matching is Critical for Up-To-Date KYC Data
Entity Extraction, Name Matching, Record Management, Risk Management
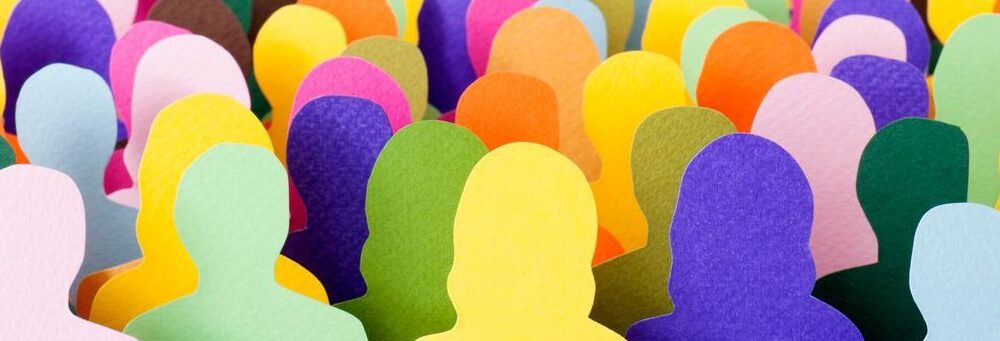
The Challenges of Know Your Customer (KYC)
KYC is a critical requirement for financial, insurance, and other institutions in order to meet regulatory requirements, serve clients better, and manage risk prudently. It is a comprehensive process to insure that a potential client can be trusted. It involves customer due diligence, risk assessment, and on-going monitoring throughout the interaction with the client.
An important step is the screening of prospective and current clients against sanctions lists produced by various organizations such as the European Union, the UN Security Council, and the US Treasury Department’s Office of Foreign Asset Control (OFAC), among many others. Individuals and organizations on those lists typically include terrorists, international drug traffickers, and targeted countries and regimes. Not meeting sanction requirements may result in substantial fines.
In addition to those official sanctions lists, companies and government organizations often want to check expanded sources to avoid doing business with parties that have been involved in illicit activities and may therefore pose a business risk.
KYC Data Providers Consolidate Available Information
Organizations looking to vet potential clients in accordance with KYC requirements have traditionally used human KYC analysts to search for information on persons and organizations in public and commercial data sources as well as their own private sources. This non-automated approach is inadequate given the very large and ever-growing amounts of data available, as well as the fluid nature of this type of information with new bad actors emerging constantly.
KYC Data Providers have stepped in to provide data from many different sources that has already been integrated and reconciled in order to provide organizations with the information needed to meet their KYC requirements.
KYC Data Providers Face a Gap in Their Coverage
KYC Data Providers have excellent coverage of many public and commercial data sources. One challenge remains, however: these data sources are inevitably going to lag behind in their coverage of recent or new adverse information about individuals and organizations such as appears in on-line media.
The solution to this challenge is to combine two advanced AI technologies, Entity Extraction and Fuzzy Name Matching.
Entity Extraction Is Critical for Mining New Data
Entity Extraction is an AI technology that is able to process very large amounts of unstructured data from media and other sources and extract the key semantic concepts:
-
- Names of persons and organizations. A critical feature of Entity Extraction is that it recognizes names of previously unknown persons or organizations. It accomplishes this through an analysis of the contexts in which these names appear in the unstructured text data, utilizing clues that indicate the presence of a name.
- Relationships among extracted people and organizations, such as employment, kinship, or subsidiary relationships
- Adverse events in which they are involved, such as bankruptcies or criminal activities such as fraud, bribery, theft, etc.
Entity Extraction also structures the extracted data for easy search and makes this extracted information verifiable by drilling down to the specific passage that conveys the information.
Using Entity Extraction, a KYC data provider is able to monitor current news stories and other media real-time, looking for relevant information such as any adverse events that companies or company leaders might be involved in.
See these links for more information on how Entity Extraction, Relationship Extraction, and Event Extraction work.
Fuzzy Name Matching Enables Intelligent Updating of a KYC Database with Newly Discovered Entities
Fuzzy Name Matching is also an AI machine learning-based technology that allows a KYC provider to compare the extracted data from recent sources with the existing KYC database built from previously available sources.
Matching extracted names against the exiting KYC database is a challenging task because names of people and organizations can vary based on many factors, including:
-
- Misspellings
- Use of a nickname vs. the full form of a name: William vs. Bill
- Presence vs. absence of an initial: John F. Smith vs. John Smith
- Names originally in a non-Latin script may be transcribed in English in different ways: Abdel Muhammad el-Sisi vs. Abdul Mohammed al-Sisi (A language like Arabic is written in a script different from Latin. When transforming the name from Arabic letters to English ones, differences in spelling frequently arise.)
- Name Order Variants: Park Jae-in vs. Jae-in Park. (Asian names place the surname, e.g., Park, first, but they occasionally occur in the Western order.)
(See this link for more examples of the challenges of Fuzzy Name Matching.)
Advanced Fuzzy Name Matching uses a state-of-the-art machine learning algorithm and large-scale, real-world name variant data. This approach automatically learns a collection of intelligent, probabilistic name matching rules from the data. Since the rules are learned from the real data, they are not bound by limitation of humans’ knowledge, but they reflect countless name variants that occur in the real world.
Using this approach, Fuzzy Name Matching will determine if newly discovered questionable people or organizations resulting from entity extraction exist in the current KYC database. If there is no match, a new person or organization record can be added. If there is a match, the record may be updated with new information and duplication of the record will be avoided.
Summary
The combination of Entity Extraction and Fuzzy Name Matching ensures that a KYC database includes the most recent information on persons and organizations that have been involved in illicit activities.
These two technologies incorporate the most advanced techniques in AI to ensure the highest accuracy and comprehensiveness.