Contact us and see what NetOwl can do for you!
How Name Matching Helps Banks Meet Their Compliance Obligations
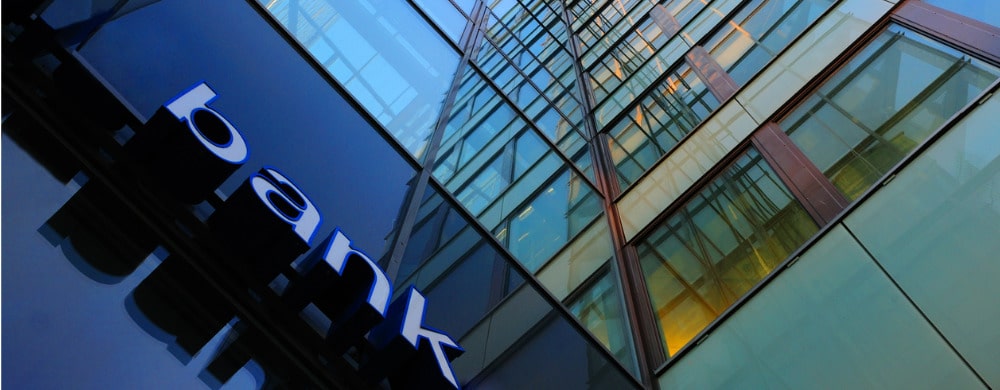
Banks Need to Modernize Their Tech Infrastructure
Digitalization is remaking all sectors of industry, and banking is no exception. Large banks in particular are dealing with outmoded technology in the face of increasing competition from the newer “fintechs.” These are companies that provide financial services and solutions with a keen focus on seamlessly integrating them into the lives of mobile device-equipped customers.
Banks are definitely behind in appealing in particular to millennials, who, just as they don’t like to go to the post office, definitely don’t want to wait in line at a bank branch or deal face-to-face with a bank employee, whether making deposits or applying for a loan or mortgage.
From another perspective, having an antiquated technology base impacts banks’ ability to attract the best tech-savvy employees. Tech workers in particular want to work with the most up-to-date stuff – it’s much more exciting and also good for their resumes.
Banks Have Compliance Obligations
One area in particular where large banks are falling behind is in one of their critical functions – that of vetting their customers and financial transactions. Banks are subject to the Bank Secrecy Act, Know Your Customer, as well as Anti-Money Laundering regulations.
Traditionally, banks have used a combination of manual and exact-match approaches, but particularly for large banks, most of which have grown through acquisitions, this produced a great deal of inconsistences in how banks met their compliance requirements. It proved difficult to standardize screenings across business units. In particular, the decision to subject an individual to further due diligence was highly idiosyncratic.
Given the lack of consistent, repeatable screening, it also proved very difficult to find hidden relationships between individuals such as one signaled by a shared physical address or the like.
How Name Matching Enables Enterprise-Level Consistent and Repeatable Screening
Name Matching, aka Entity Matching, is an AI technology that enables strong and reliable KYC and AML compliance. With it a bank can screen prospective and current customers against sanctions lists maintained by such institutions as the US Treasury Department’s Office of Foreign Assets Control (OFAC), the European Union, and the UN.
Data that a bank obtains from a customer typically consists of multiple fields, such as personal name, social security number, home address, phone number, etc. Multi-field Name Matching applies fuzzy name matching specifically tailored to each field, which is probabilistic matching that calculates the distance between one data record and what is contained in the sanctions lists.
Each field has its own variant characteristics that Multi-field Name Matching handles:
- Personal names may vary in multiple ways, such as:
- Nicknames: Thomas vs. Tom vs. Tommy; Mikhail vs. Misha; Alexandra vs. Sasha
- Use (or the absence) of middle names or initials : John Frederick Stone vs. John F. Stone vs. John Stone
- Differences in ordering of name elements: William Randolph Hearst vs. Hearst, William Randolph
- Also, due to the great ethnic diversity in the U.S. and increasingly globally, there are names of many different ethnic types, which have their own unique variations. Here are just a few of them:
- Spanish names frequently contain both patronymics and matronymics (surnames from the father and mother, respectively); alternatively, one of them (matronymics) may be dropped: Miguel Esteban vs. Miguel Esteban Ramos.
- Foreign languages in Latin script also frequently contain diacritics, which may be absent: Joaquín Guzmán vs. Joaquin Guzman
- Arabic names, which commonly contain an element “al-,” may appear with or without it, as in Mahmoud al-Badawi vs. Mahmoud Badawi
- Some East Asian names are in the last name + first name order while others are not: Xi Jinping, Moon Jae-in, but Shinzo Abe
- Dates also exhibit multiple variations, e.g.:
- The ordering of the component parts of dates may vary:
- 9/23/2014 vs. 23/9/2014 (US Month-Day-Year vs. UK Day-Month-Year)
- Month or Day Names may be spelled out, abbreviated, or numeric:
- November vs. Nov., Monday vs. Mon.
- The ordering of the component parts of dates may vary:
- Likewise addresses, e.g.:
- Elements may be spelled out or numeric:
- Ten Williams Drive vs. 10 Williams Drive
- Frequent abbreviations:
- Road vs. Rd.
- There may be multiple differences in one address:
- 6901 7th Street, Scarsdale, NY vs. 6901 Seventh St., Scarsdale, New York
- Elements may be spelled out or numeric:
Name Matching matches all of these different types of fields successfully. It first matches each field separately and produces a similarity score for it. It then combines the scores of each field into a single overall score that indicates the probability that one customer record is matching an entry in the sanctions lists. Some fields may be weighted more depending on the business logic.
Since sanctions lists such as OFAC frequently contain data on individuals and organizations that go beyond just names, such as dates of birth, places of birth, addresses, phone numbers, it’s critical to use Multi-field Name Matching to make good matches using all the available fields.
How Name Matching Works
Name Matching enables accurate matching and also the high throughput that is required by a large bank to meet its compliance obligations. Under the hood, Name Matching employs AI-based machine learning algorithms that create a distinct matching model for each entity type such as person, organization, and place. These models are trained to match the likely variations that occur within that type. Training is accomplished using a very large amount of real-world data.
In sum, banks can get a real leg-up in the increasingly digitized marketplace through using Name Matching.