Contact us and see what NetOwl can do for you!
How Entity Extraction Can Provide the Missing Links for Financial Intelligence
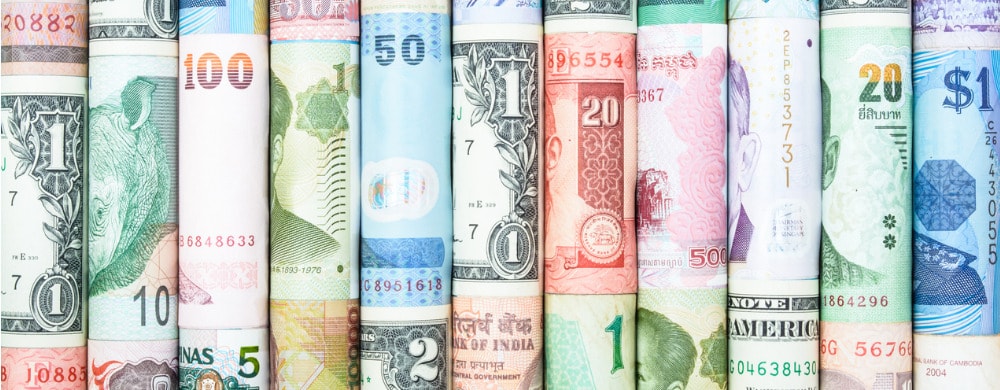
What Is Financial Intelligence?
Financial Intelligence (FININT) is the collecting and analyzing of financial information on entities of interest in order to uncover criminal activities (e.g., money laundering, tax evasion, terrorist financing). Examples of the results of Financial Intelligence analysis are:
- Finding connections between terrorist cells through remittances
- Understanding the banking networks being used for money laundering
- Uncovering corruption.
With financial crimes becoming increasingly global and complex, Financial Intelligence has gained critical importance and is critical to discovering criminal behaviors involving complex networks.
How Entity Extraction Can Improve Financial Intelligence
Entity Extraction helps analysts get on top of enormous volumes of data to achieve the following goals:
- Recognizing criminal actions
- Unearthing hidden links between accounts
- Tracking relationships between actors engaged in criminal activity
Although much of the data is structured transactional data (e.g., inter-bank transfer data), a large and growing amount of relevant data is coming in the form of unstructured data, specifically text data. Examples of such data include:
- Suspicious Activity Reports that are required of banks by the U.S. Government when they identity potentially criminal activity involving money transfers
- Crime reports
- News reports
- Email messages
- Social media.
Advanced text analytics technologies like Entity Extraction can expedite the processing and review of unstructured data and free up analysts to do more complex analytical work. It can also facilitate the discovery of hidden connections between pieces of critical information from seemingly unrelated data sets.
How Does Entity Extraction Work to Develop Financial Intelligence?
Entity Extraction begins by extracting the basic building blocks of information from unstructured text data: named entities.
Entity Extraction doesn’t just extract names that are already known to an analyst seeking pertinent information. It finds all names, including more importantly names that the analyst has not encountered before. Entity Extraction also categorizes the names: it classifies the names whether they refer to people, organizations, products, and many other types. In this way Entity Extraction automatically builds an index of names that gives immediate insight into the contents of documents.
Even just with extracted names, a financial analyst can gain a rich source of new information. For example, an analyst can issue queries like “Show me all names of individuals ranked by frequency of occurrence in the document set.” In addition, an analyst can study the co-occurrence of individuals and organizations.
Relationship Extraction Finds Associations among Entities
Relationship Extraction goes a step beyond the extraction of named entities. It focuses on identifying relationships between entities. It is superior to simple statistical co-occurrence as it indicates the semantic nature of the relationship between two entities. For example, it identities the following types of relationships critical to developing Financial Intelligence:
- a person’s associates
- a person’s affiliations
- an organization’s subsidiaries
Relationship Extraction extracts a set of pre-defined semantic relationships that permit the many different ways of expressing the same relationship to be normalized into a single structured output format. This permits the easy aggregation of the data.
One popular use of such structured output is to populate a link analysis tool that visually displays all the relationships extracted from unstructured data, for instance, for social network analysis.
Event Extraction Identifies Events in which Entities Have Participated
Event Extraction is also of great help in collecting Financial Intelligence. This extracts what happened, along with the Who, What, Where, and When of the event. For example, Event Extraction identifies adverse events in the news media such as crimes, arrests, indictments, etc.
Once entities, links, and events have been identified, the information can be exploited not only by more advanced semantic search but also by link analysis and other knowledge discovery tools.
All in all, Entity Extraction enables a deep analysis of unstructured data and uncovers critical information and insight for Financial Intelligence.