Contact us and see what NetOwl can do for you!
How Entity Extraction-Based Redaction Unlocks EHRs while Protecting Patient Privacy
Entity Extraction, Intelligence Analysis, Record Management, Risk Management
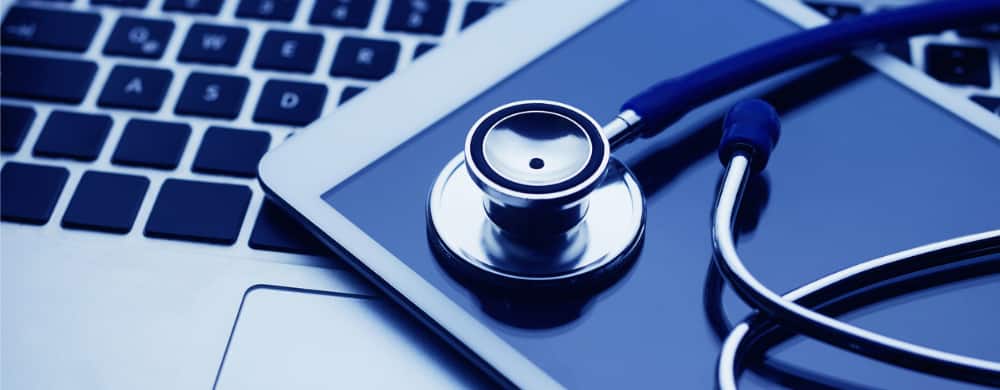
Electronic Health Records (EHRs) Allow Easy Sharing of Health Information
Data digitalization has been of revolutionary importance for many industries, but perhaps none more so than in health care. It has opened the way for the adoption of Electronic Health Records (EHRs), which have made it possible for clinicians and researchers to gain access to and perform research on extremely large amounts of patient data and to inform evidence-based decision making. It has also enabled the seamless sharing of such data with other health care providers that also require the data as well as with third-party organizations such as insurance companies that may have a legitimate need to access relevant data.
EHRs Create Some Risks to Patient Privacy
The downside to all of this is that in some ways patient privacy was better protected in the days of paper records. The latter were restricted to folders in file cabinets, and it was cumbersome to share them. Now patient-sensitive information can be shared instantaneously via networks within medical institutions and even across the internet. EHRs contain a very large amount of sensitive data about individuals: a typical medical history will contain Personally identifiable information (PII) data such as name, address, phone number(s), email addresses, etc., and will also contain medications being taken, diagnostic reports from physicians, details on alcohol and recreational drug use, pre-existing conditions, allergies, surgeries undergone, etc.
As a response to privacy concerns, the Health Insurance Portability and Accountability Act (HIPAA) was passed in 1996 by the U.S. Congress. HIPAA created standards for the protection of patients’ medical records and other personal information. It covers, among other actors, health care providers and health plans that handle transactions electronically. Its Privacy Rule requires redaction of sensitive information elements.
Redaction of Sensitive Information Used to Be Done Manually
The traditional way of performing redaction of sensitive information was to manually black out the sensitive terms prior to a document’s release. This manual process may be sufficient when the number of documents to be redacted is small. But the large volumes of EHRs call for an automated solution that can identify sensitive information accurately, robustly, efficiently, and at scale. Fortunately, there is a technology available that can do this: Entity Extraction.
How Entity Extraction Helps Redact Medically Sensitive Information
Entity Extraction (aka Named Entity Extraction or Named Entity Recognition) identifies important concepts in unstructured text. These include PII such as names of people, Social Security Numbers, driver license numbers, dates of birth, phone numbers, home addresses, email addresses, etc., all of which need to be redacted. It doesn’t recognize them just by having a big dictionary of these concepts. Rather, it uses the surrounding context to identify clues that suggest, for instance, the presence of a name. In other words, Entity Extraction’s most important contribution is that it identifies concepts in a dynamic fashion – it recognizes instances of names it has not seen before. For example, it will not only recognize that “John Richardson” is a name and refers to a person, but it will also recognize that a previously unseen string like “Pao Bangfu” is also a person name based on context.
Entity Extraction identifies both full names (e.g., “John Richardson”) and partial names (e.g., “Richardson”, “John”, “John R”). Furthermore, Entity Extraction identifies name mentions referring to the same person. This is useful when a medical record mentions multiple people (e.g., patient, medical staff, relatives). If all person mentions are redacted the same way (e.g., replaced with the same generic label like <PERSON>), the resulting medical record could be confusing to read. If instead the medical record can be redacted more intelligently (e.g., with a different label for each person like <PERSON1>, <PERSON2>, <PERSON3>), the resulting redacted document will be more intelligible and useful.
Entity Extraction can distinguish PII from other concepts that look similar but should not be redacted. For instance, Forbes, Albright, Andersen, Flint, Wharton, and Mitchell are common last names, but should not be redacted when referring to syndromes (e.g., Forbes-Albright syndrome), diseases (e.g., Andersen disease), medical signs (e.g., Austin Flint murmur), anatomical parts (e.g., Wharton’s duct), treatments (e.g., Mitchell’s rest cure), etc.
Entity Extraction automatically identifies all entities such as names of people in unstructured text very quickly and accurately. When paired with a human redactor for verification (in a so-called human-in-the-loop setup), the human redactor is enabled to quickly focus on precisely those portions of a large text that contain the relevant concepts.
In sum, Entity Extraction offers a fast, accurate, and economical way to redact the sensitive information in medical reports to unlock critical information for researchers and evidence-based decision making.