Contact us and see what NetOwl can do for you!
Entity Extraction: The AI Key to Unlock Your Unstructured Big Data
Categorization, Enterprise Search, Entity Extraction, Geotagging, Intelligence Analysis, Sentiment Analysis, Social Media Analysis
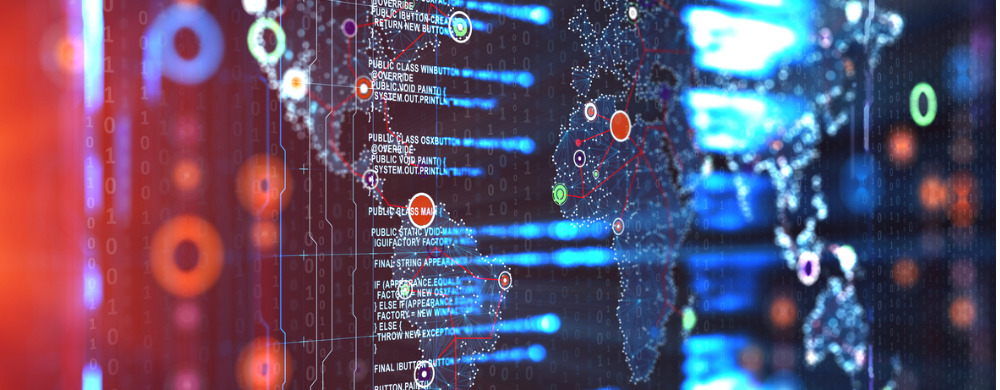
So you have tons of unstructured text data, maybe terabytes or even petabytes. It may be that your data comes from a variety of sources such as news, social media, email, reports, files in various proprietary formats, or even OCR output. And it may be that it includes a mixture of languages.
You know that there is much value in your data, but its sheer size and complexity is daunting and it seems all you can do is make it keyword searchable. You know there are big-picture trends waiting to be discovered along with individual high-value nuggets of information, but how can these be discovered without having to read all of that content?
Your past experience with search allows you to find some relevant information of interest from the collection, but you still find yourself having to read lots of individual documents to really discover key insights. You may have tried some open source tools to help bring forth such information, but were disappointed by their performance, perhaps because of poor accuracy, limited capabilities, or inadequate throughput.
This is a very common scenario that our customers come to us for. Based on years of experience working on this type of problem with customers in various markets and industries, in both the public and private sectors, we at NetOwl have a solution for you.
Comprehensive Text Analysis
Let’s start at the very beginning. Once the data has been collected, or as it comes in, each data source provides some basic document-level metadata that can be used to help restrict attention to particular data sets or time ranges of interest. NetOwl Extractor however allows you to tackle the actual text content to help uncover the knowledge it contains. First, if the data is multilingual, NetOwl Extractor can identify the language(s) in the document to determine the optimal language configurations to use when processing each individual text. Based on the identified languages, NetOwl applies its core text analytics capability: Entity Extraction.
All language modules, ‘configurations’ in our terminology, share the same core ontology. An ontology is a semantic hierarchy that determines what concepts NetOwl will look for in text. NetOwl offers a broad and deep ontology that goes beyond the standard named entity ontology offered by other open source or commercial products. At the entity level, it spans over 100 different types of entities (e.g., people, various types of organizations, places, addresses, and artifacts). At the more advanced level, it discovers over 150 types of relationships and events that connect the entities together, a unique capability in the Text Analytics space. Superset ontologies are available such as for the cyber security domain that identify additional concepts (e.g., malware, cyber attacks). At any level of extraction, custom concepts may be added through the Creator Edition.
Sentiment Analysis can be performed to capture the opinions, likes, dislikes, and intent expressed in your data. Sentiment Analysis has multiple applications. In the commercial sector, it is used for brand monitoring and protection, voice of the customer, market research, digital marketing, etc. In the public sector it is used for situational awareness (e.g., aftermath of a natural disaster) and public opinion gauging (e.g., public reaction to a public health campaign or to a proposed policy change), among others. Unlike simple sentiment analysis tools that identify positive and negative language, NetOwl’s Entity- and Aspect-based Sentiment Analysis pinpoints the specific entity or entity aspect that the sentiment is about. People may find that a product has some great features but it’s expensive. Identifying the nuances of what the sentiment is really associated with can be of great value.
Another useful text analytics technology is Geotagging. NetOwl’s Smart Geotagging accurately assigns latitude/longitude values to place names as well as to other geocodable entities and events extracted from text. First, it recognizes when an ambiguous name like ‘Paris’ refers to a place rather than a person or something else. Then, it uses context to decide what Paris is being talked about: is it Paris, France or Paris, Texas? Once place names are geotagged, any entities associated with that location like a person visiting or an event taking place there can also be geotagged. With NetOwl Smart Geocoding, you can now visualize your unstructured documents on a map providing context of who was there, what they did, and when it happened.
Separately, state-of-the-art categorization may be used to classify your dataset according to any set of out-of-the-box or user-defined categories. For maximum flexibility, a multi-strategy approach allows for Machine Learning-based, rule based (topic-based), and extraction-based categorization.
Advanced Visualization and Analytics
As texts are processed in real-time or in batch mode, NetOwl can represent the extracted information in a variety of formats (e.g., JSON, XML, RDF) depending on what fits best with your overall document processing workflow. Often NetOwl’s structured output is stored in a repository, usually a NoSQL database like Elasticsearch, Accumulo, or Mongo DB, and the results are exploited through a search, geospatial, or business intelligence tool that is connected to the repository.
NetOwl’s analytical tool TextMiner offers a variety of intelligent search and analytic capabilities both to examine the data in aggregate and to drill down for further knowledge discovery. Here are some of the ways you can analyze your data with TextMiner:
- Faceted search: facets are semantic filters that you can use to browse and discover the entities and events extracted from your data. You may start with a specific facet (e.g., a company or person of interest) and add other facet values based on the extraction results (e.g., merger events) to narrow down your document search and extraction results. Faceted search not only can help you refine your search, but can be used to discover the most frequently mentioned extracted entities and events associated with any type of document search performed in TextMiner as a form of knowledge discovery.
- Frequency-based analysis: frequency-sorted lists of entities and events provide a great sense of the topic nature of a document set or subset and the topic trends therein.
- Timeline analysis: a timeline allows you to see volume over time and immediately spot spikes in volume for an entity of interest (e.g., a foreign leader). For instance, given a Twitter feed, the user can select a given spike to narrow down the data to that time segment for further inspection.
- Profile/biography generation: automatically generated biographies of a person, organization, place, or other entity types allow you to see information aggregated from a large collection of documents. A person profile may contain information such as aliases, age, titles, family members, associates, affiliated organization, etc. An organization profile may include its key executives, subsidiaries, headquarters locations, etc.
- Event View: you can inspect not just any number of events expressed in multiple ways but also their participants (e.g., perpetrator, victim, weapon, time, place).
- Geospatial analysis: TextMiner’s map view shows not only the geotagged place entities associated with a document set but also the entities and events that are semantically linked to a geotagged place providing visual evidence of more interesting context to each specific location. For example, a map may show icons for bombing events or people that are connected to that location and can link those specific associations to the original source documents.
- Link Graph: given an entity, TextMiner’s link graph view displays all the semantic relationships that the given entity has with other entities. You can grow this initial network of connections step by step by selecting any of the linked entities on the graph.
- Sentiment dashboard: TextMiner presents multiple views of the sentiment information through various types of interactive graphs and charts. Sentiment data can be sliced and diced as desired, for instance by positive and negative aspects (e.g., price, customer service), and you can drill down to the source text for inspection and further analysis. Other useful charts show sentiment evolution over time through a sentiment timeline.
NetOwl’s best-of-breed Entity Extraction along with advanced text analytics capabilities enables you to perform deep analysis and visualization of your Big Data. To see all of this in a live demo, contact us!